Machine learning in banking and finance: opportunities, risks and use cases
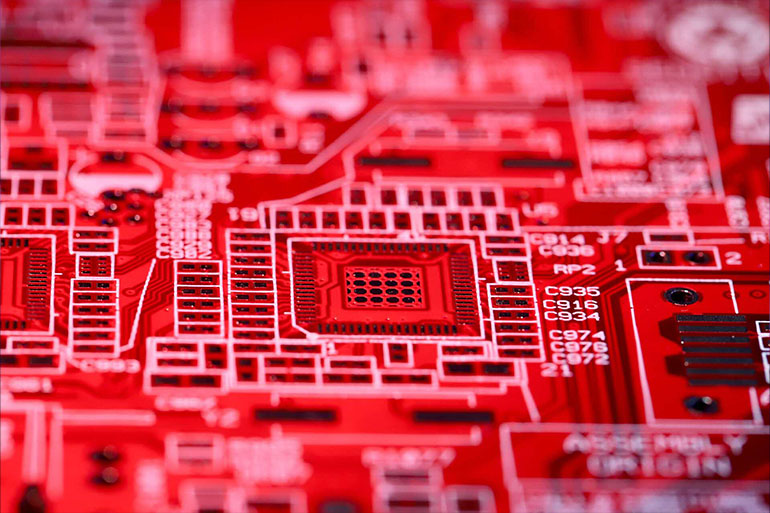
25 Oct 2023
Machine learning, or ML, is revolutionising numerous industries, including the banking industry and financial services sector. When it comes to machine learning in banking, leading financial institutions are harnessing ML's capabilities to streamline operations, enhance customer experience, bolster risk management, and improve financial forecasting.
In this article, we delve deeper into the application of machine learning in banking and for finance, exploring its opportunities, potential risks, use cases, benefits, and pivotal role in improving real-time fraud detection.
Machine learning in banking and finance
To understand machine learning better, here are some examples of real-world machine learning models and algorithms in banking and finance.
Sifting through big data manually can take a lot of human effort and time. With the help of machine learning, a computer program that looks at this data can quickly identify patterns and automatically categorise this data – even making predictions or recommendations.
For example, machine learning can identify financial habits of a certain demographic, or flag potentially risky transactions that could be linked to fraud or scams to a bank’s compliance team. In addition, the time saved with automation can be better used on strategic analyses or quality customer interactions.
In which areas of banking/ finance is machine learning used?
The following is a summary of some of the ways machine learning is being used in, and how it impacts/ benefits the banking and finance industry:
- Quicker fraud detection and prevention through data analysis and detection of anomalies.
- Enhancing traditional credit scoring models that can consider a broader range of data.
- Helping financial institutions assess and manage risk more effectively.
- High-frequency algorithmic trading that can analyse market data, execute complex trading strategies and react to market conditions in real-time.
- Customer service and chatbots powered by natural language processing (NLP) and machine learning that help to answer high volume routine queries.
- Personalised banking, where offerings are tailored to better meet customers’ individual needs and preferences.
- Automating compliance tasks by analysing and categorising vast amounts of legal and regulatory documents.
- Anti-Money Laundering (AML) and Know Your Customer (KYC) compliance and spotting potentially illicit transactions and suspicious activities.
- Predictive analytics that can forecast financial trends, customer behaviour, and market conditions to inform business strategies and investment decisions.
For instance, DBS NAV Planner, a financial planning tool that aims to make financial advice easily accessible for our customers, comprises a suitability engine. The AI/ML models behind this tool are trained to recommend the right investment products to the right people, while also figuring out the best advice to give customers based on their individual profile.
How do banks benefit from machine learning?
With the above non-exhaustive use cases, it is seen that banks can benefit significantly from machine learning in various aspects of their operations, allowing them to enhance customer experiences, improve risk management, streamline operations, and drive revenue growth. Here’s a deeper dive into some areas of note:
Customer experience enhancement: machine learning algorithms enable banks to analyse customer data and behaviour (taking into consideration data protection and privacy laws) to provide personalised services and recommendations.
Credit risk assessment: machine learning models help banks assess credit risk more accurately. They analyse vast datasets to identify patterns and predict the likelihood of loan defaults. By employing machine learning, a bank can make more nimble and more informed lending decisions while minimising risk and improving the quality of their loan portfolio.
Operational efficiency: automation through machine learning reduces the need for manual intervention in routine banking processes. For instance, DBS Bank employs chatbots to assist customers with common inquiries and tasks, allowing human staff to focus on more complex issues. This improves operational efficiency and lowers costs.
Revenue generation: machine learning could also help banks identify cross-selling and upselling opportunities. By analysing customer data, banks can recommend tailored financial products and services to their clients, whether via nudges or through a recommendation engine.
DBS has leveraged machine learning to our advantage. We embarked on our digital transformation journey early in 2014, well ahead of our peers. In 2022, our AI/ML use cases delivered an economic value of SGD 180 million, comprising a revenue uplift of SGD150 million as well as SGD30 million from cost avoidance and productivity gains.
Opportunities of machine learning in banking and finance
Machine learning in banking offers immense opportunities. One of the most significant lies in understanding customer behaviour, which can improve customer experience. According to Harvard Business Review, machine learning holds much promise. Why? Because ML can predict customer behaviours.
The article notes, “By deploying ML to predict which content is most relevant for each individual, customers can receive better recommendations, less junk mail, very little inbox spam, and higher quality search results, among many other things. These improvements to customer experience aren’t only a nice-to-have, pleasant side-effect of profit-driven ML deployments. They pursue the raison d’etre of any company – to serve customers – and will ultimately translate into further benefits for the business. After all, a happier customer is a more loyal customer, and a higher customer retention rate means a higher customer growth rate.”
At DBS, we’ve created more than 600 artificial intelligence (AI) and machine learning models that can, for instance, offer personalised product recommendations and celebrate our customers’ milestones. Today, our Consumer Banking Group sends out 45 million hyper-personalised nudges monthly to over five million customers across the region to guide them towards better investment and financial planning decisions.
Risk management is another area where machine learning can make a substantial impact. The book, Disrupting Finance: FinTech and Strategy in the 21st Century, notes that the areas of consumer lending and small- and medium-sized enterprises (SME) lending involve large amounts of potential data and are increasingly relying on machine learning to make better lending decisions. The same goes for machine learning in managing market risk, and operational risk.
DBS has been a frontrunner in using ML for risk management. AI/ML enables us to pro-actively provide SMEs with early alerts of credit risks, allowing SMEs to take pre-emptive action and nip potential credit issues in the bud. In 2022, we were able to successfully identify over 95% of non-performing SME loans at least three months before the businesses experienced credit stress. Over 80% of identified at-risk borrowers were averted from risk.
Risks of machine learning in banking and finance
Despite the numerous benefits that come with machine learning for the banking and finance sectors, this is not without risks. One of the main challenges of utilising ML is ensuring the privacy and security of customer data as banks increasingly rely on ML algorithms to process and analyse customer information.
EY notes that “AI/ML early adopters face increased risks, such as lawsuits arising from the use of web-based copyrighted material in AI outputs, concerns about bias, lack of traceability due to the ‘black box’ nature of AI applications, and threats to data privacy and cybersecurity”.
For instance, while machine learning models are powerful, they are only as good as the data they are trained on, which may be biased or incomplete. Meanwhile, the lack of transparency and interpretability in some machine learning models (‘black boxes’) make it difficult for humans to understand how they make predictions.
At DBS, we implemented the PURE Framework – which assesses data use cases against PURE Principles (Purposeful, Unsurprising, Respectful, Explainable) – to ensure responsible data use across the bank. This has been further enabled through the development of an in-house assessment tool and overlaid with formal governance procedures, operating models, and the introduction of mandatory training.
Can machine learning improve fraud detection in real time?
Machine learning is revolutionising the way banks detect and prevent fraud. According to research, ML techniques have been applied very effectively to the problem of payments related fraud detection, and have the potential to evolve further, to detect previously unseen patterns of fraud. Even outside of banking and finance, ML has been used to detect potentially fraudulent activity, such as for Amazon Web Services credit card transactions.
The problem with traditional fraud detection systems is that it often struggles to keep up with the increasingly sophisticated tactics employed by fraudsters. However, machine learning models that are trained well can analyse transaction data in real-time, identify anomalous patterns, and flag potentially fraudulent activities.
At DBS, we have a dedicated anti-scam team with round-the-clock response capabilities to carry out intervention work, monitor and review fraud alerts. We carry out transaction screening with our monitoring and surveillance systems, which helps us detect unauthorised activities on customer accounts. With the aid of AI/ML, we can detect distinct changes in user behavioural patterns; cross-reference unusual account changes; as well as instantly screen and block flagged transfers.
From June to July 2023, DBS worked with the Singapore Police Force, helping to disrupt more than 1,400 active scam cases. With the help of ML, we automated processes to significantly reduce the time taken to identify scam victims. We also leveraged technology to automate information sharing, information processing and the mass distribution of SMS alerts within a short time, improving outreach.
In the same vein, by adopting machine learning for finance, banks can enhance their fraud detection capabilities, reduce false positives, and react swiftly to suspicious activities, thereby minimising financial losses and maintaining customer trust.
In conclusion, machine learning in banking holds immense potential. As financial institutions continue to invest in this technology, we can expect to see further advancements in the way banks operate, manage risks, and serve their customers. However, it's crucial for banks to address the associated risks, particularly around data privacy and security, to fully harness the benefits of machine learning.